Insights Engine is an intelligent search service powered by machine learning with which enterprises can easily find the content they are looking for, even when it’s scattered across multiple locations and content repositories within your organization. It reduces the cost, effort and errors involved in looking for the data manually. Using Insights Engine, you can discover the right answers to your questions when you need them, without having to scavenge through heaps of unstructured data. Insights Engine is a complete, managed service, so there are no servers to provision, and no machine learning models to build, train, or deploy.
What is “Active Learning”?
Most supervised machine learning models require large amounts of data to be trained with excellent results. And even if this statement sounds naïve, most companies struggle to provide their data scientists with this data, in particular labelled data. The latter is key to train any supervised model and can become the main bottleneck for any data team.
Active learning is the name used for the process of prioritizing the data, which needs to be labeled in order to have the highest impact on training a supervised model. Active learning can be used in situations where the amount of data is too large to be labeled and some priority needs to be made to label the data in a smart way.
Insights Engine answers users’ queries by showing relevant responses from the documents. The responses are ordered in decreasing order of confidence match. But the biggest challenge is how do we train our model to learn from user feedback and improve search performance over time?
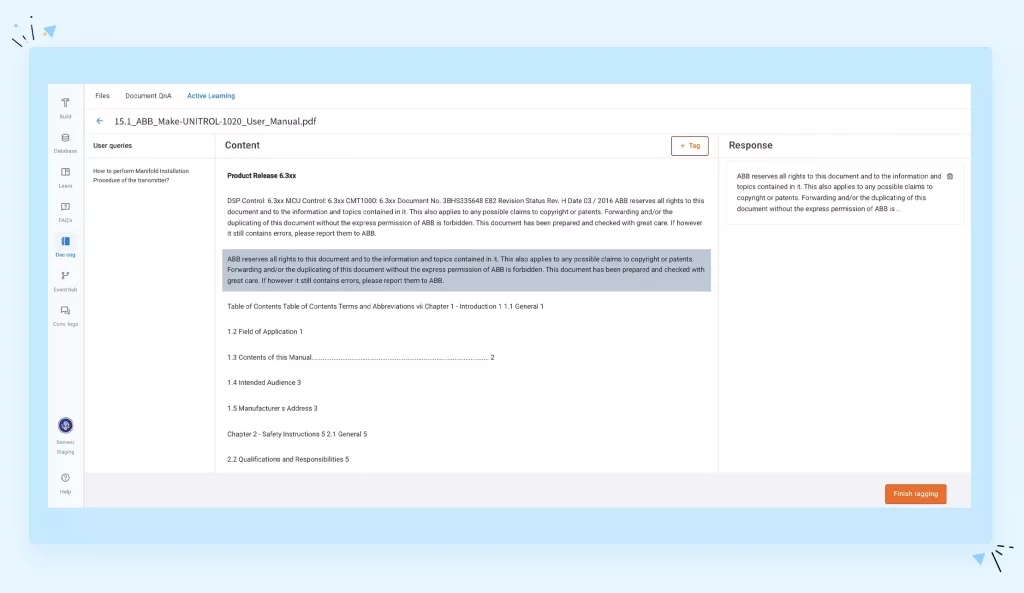
The user can choose a group based on its performance and review the queries inside that cluster. Users can either review one query at a time or a group of queries together that the user finds similar.
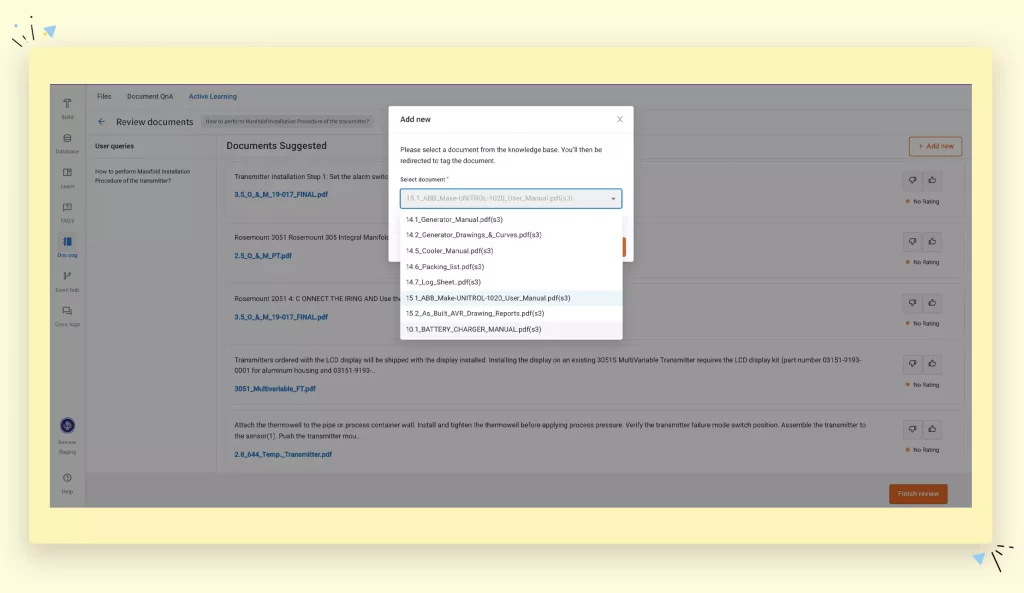
Once the user wants to review, all search results appear for the query/queries.
The user can either train the model by submitting the feedback in form of a thumbs up/thumbs down or tag a new response from the document that he wants to show in the search results.
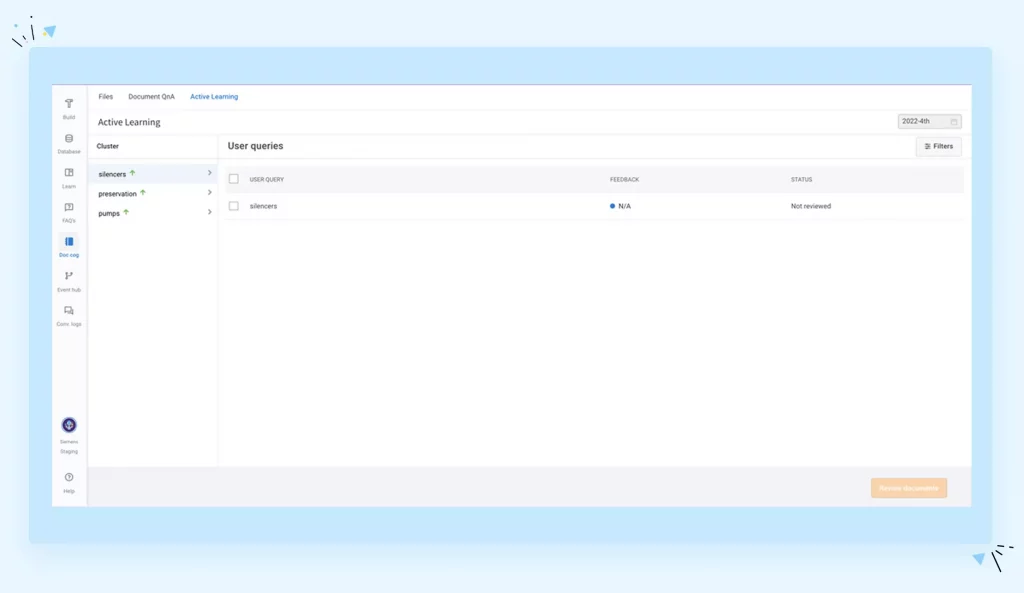
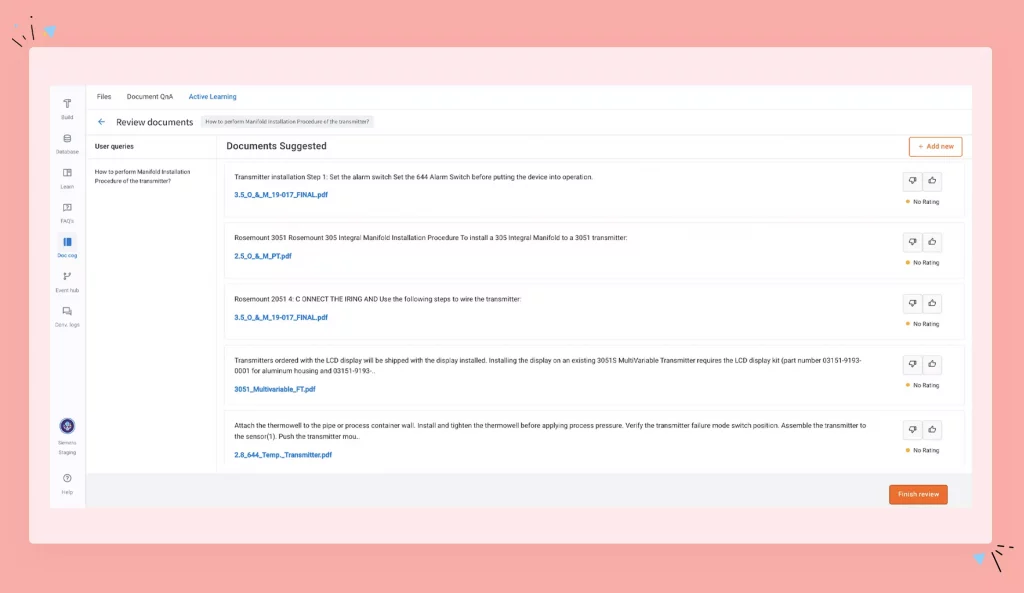
The model incorporates this training in the model and improves search performance over time.
Watch this demo video to learn more about active learning :
With active learning, the overall accuracy and quality of conversations are enhanced.
– It helps improve search accuracy and relevancy of results
– The feedback on model performance is faster.
– Data labeling becomes highly efficient.
– It works best as the audience and user base of businesses expand.
– It helps to fill any gaps in the knowledge base.
– The chatbot software is capable of conducting an enriched conversation with users.