In the era of digital transformation, customers have come to expect 24/7 omnichannel services from companies. Enterprises need to keep pace with these demands by providing personalized, automated self-service support.
Traditional chatbots are widely used in organizations and companies to cater to these ever-evolving customer needs. However, a common complaint from customers who communicate with bots is that they sometimes fail to grasp the context of conversations. Organizations can use AI chatbots & voice bots, also known as conversational AI, to solve this problem.
Conversational AI enhances the customer experience by assisting in developing chatbots that are more intelligent and proficient at comprehending and responding to human language. Unlike traditional chatbots, AI chatbots are not rule-based; they are trained by engaging in millions of conversations and continue to learn from each conversation.
In this guide, we’ll start by explaining what conversational AI is and how it works. Then we’ll discuss different types of conversational AI, potential benefits, and challenges of implementing this technology into your customer experience. Lastly, we’ll explore real-life conversational AI examples and walk you through how your organization can get the most out of this technology.
To start with the basics. let’s define conversational AI and dive into its four key components.
What is conversational AI?
Conversational AI technology enables humans to have conversations with machines that feel like they are talking to another person. To achieve this, conversational AI uses various technologies like machine learning (ML) and natural language processing(NLP). As more people use these bots, they become smarter and better at understanding what people mean and predicting what they might say next.
Four components of conversational AI
1. Machine Learning (ML)
Machine Learning (ML) is a branch of artificial intelligence that employs a variety of algorithms and statistical models to identify patterns and make predictions. Machine learning is a critical element of conversational AI. It enables the system to continuously learn from the data it gathers and enhance its comprehension of and responses to human language. Conversational AI frequently employs supervised learning, unsupervised learning, deep learning, and neural networks, among other ML subtypes.
2. Natural Language Processing (NLP)
Natural Language Processing (NLP) involves transforming unstructured data into a machine-readable format and processing it to produce an appropriate response. These NLP techniques engage in a continual feedback loop with machine learning, enabling continuous improvement of the algorithms that are the building blocks of conversational AI.
NLP is essential to conversational AI since it allows the system to comprehend user input and produce relevant responses. It consists of four main processes to comprehend human language:
- Input generation: Input generation is the process of producing fresh input for a conversational AI system. Users can provide input through a website or an app as text, speech, or both.
- Input analysis: Intent analysis determines the meaning and intent of a user’s input. If the input is text-based, conversational AI will use natural language understanding (NLU) to decipher the content of the input and ascertain its purpose. In the case of voice input, it will employ a mix of automatic speech recognition (ASR) and natural language understanding (NLU) to decipher the message.
- Dialogue management: Dialogue management controls the conversation’s flow, including whether to clarify or take a pause. This function is formed by Natural Language Generation (NLG). Conversational AI uses dialogue management to track conversations and determine what information has already been received and is still needed. As a result, the system can continue the conversation by asking questions or offering more details.
- Reinforcement learning: Reinforcement learning is training a system to make independent decisions by rewarding or punishing specific behaviors. The system is given an objective, and it employs a variety of strategies to complete the task. It obtains a score for each decision it makes depending on how efficiently it helped it complete the mission. It is used in NLP to accept corrections and learn from its experiences to provide a better answer in subsequent interactions.
3. Data mining
Data mining is extracting usable information from enormous amounts of data. Data mining is used in conversational AI to discover patterns and insights from conversational data that developers can utilize to enhance the system’s functionality. Although it has many traits in common with machine learning, data mining is a method for identifying unknown properties as opposed to machine learning, which focuses on generating predictions based on recent data.
4. Automatic speech recognition (ASR)
Automatic speech recognition is a component of AI for voice-based conversations. ASR enables the system to understand human voice inputs, filter out the background noise, use speech-to-text to deduct the query, and simulate a human-like response. There are two types of ASR software – Directed dialogue and natural language conversations.
Directed dialogue is a simpler version of ASR that can answer basic yes/no questions. Natural language conversations are more complex and improved versions of ASR that simulate actual human conversations.
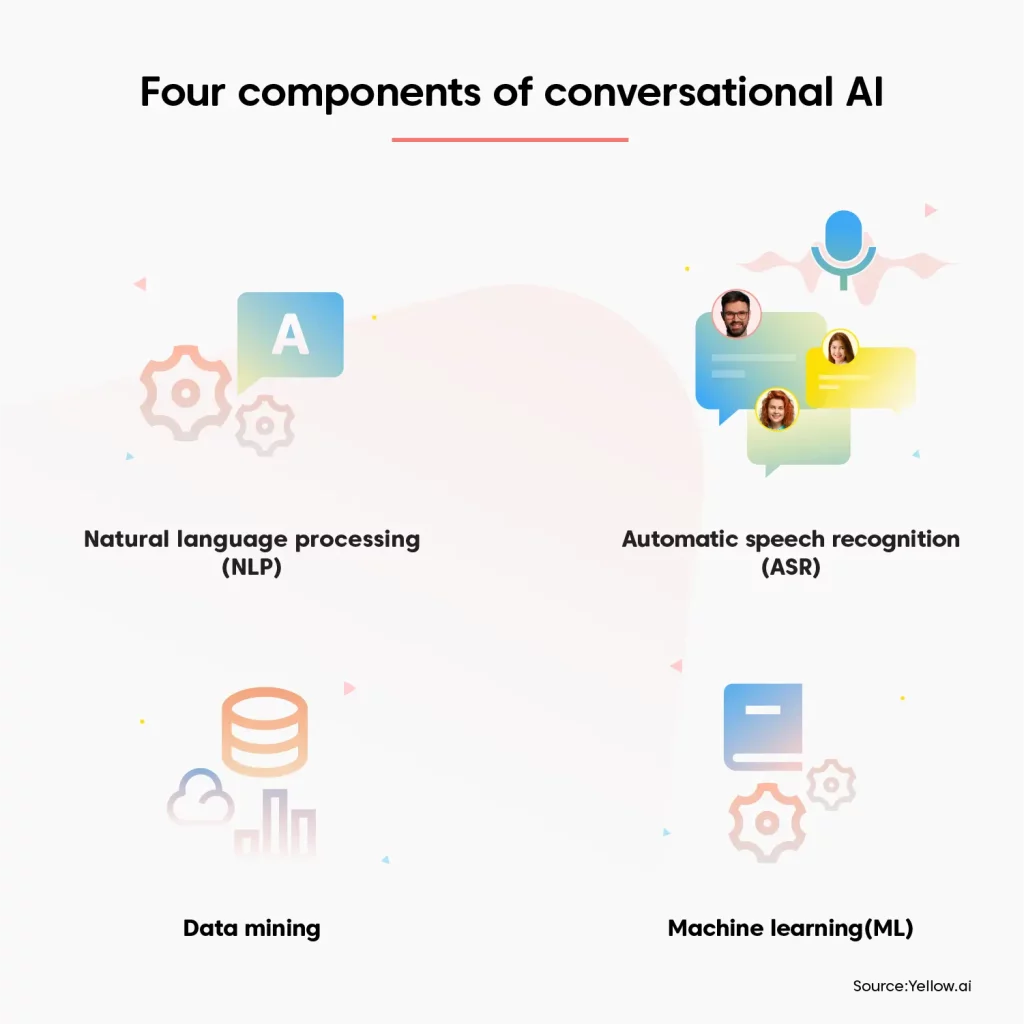
How does conversational AI work?
Conversational AI combines a variety of technologies to understand, react and learn from every encounter, including automatic speech recognition (ASR), natural language processing (NLP), advanced dialogue management (ADM), and machine learning (ML).
NLP and interpreting user input
Understanding user input is the first stage in conversational AI. NLP enables the system to examine human input and derive meaning from it. The system employs several NLP methods, including tokenization, parsing, and semantic analysis, to comprehend the structure and intent of user input.
Dialogue management and context tracking
After the system has comprehended the user’s input, dialogue management tracks the conversation’s context and establishes the user’s intent. This enables the system to comprehend the user’s requirements and respond correctly. Additionally, the system uses context monitoring to keep the dialogue on course and comprehend the user’s current requirements.
Recognizing user intent and figuring out their objectives
The following stage establishes the user’s intention or objective based on the user’s input. Once the request has been created using NLP, a variety of deep learning and machine learning models take over. Natural language understanding (NLU) refers to a set of techniques that allow conversational AI to determine the correct intent (or topic) of a request and extract additional information that can be used to trigger additional actions, such as context, account preferences, and entity extraction.
Generating a response using TTS or text responses
Once the user’s intent has been identified, the system responds. The system uses dialogue management to build the answer based on its comprehension of the text’s intent. Natural language generation (NLG), another element of NLP, orchestrates the responses and converts them into a human-understandable format.
The application sends the response in text or utilizes speech synthesis or text-to-speech (TTS) to convey it over a voice modality.
Continuous learning and improvement through user data and feedback
The third phase is continually learning from user input and data and enhancing the system. The system utilizes machine learning techniques to learn from user interactions and improve over time. This enables the system to improve its accuracy and effectiveness gradually.
Types of conversational AI
Different varieties of conversational AI have different capabilities, strengths, and limits. Let’s examine a few of the most common types of conversational AI.
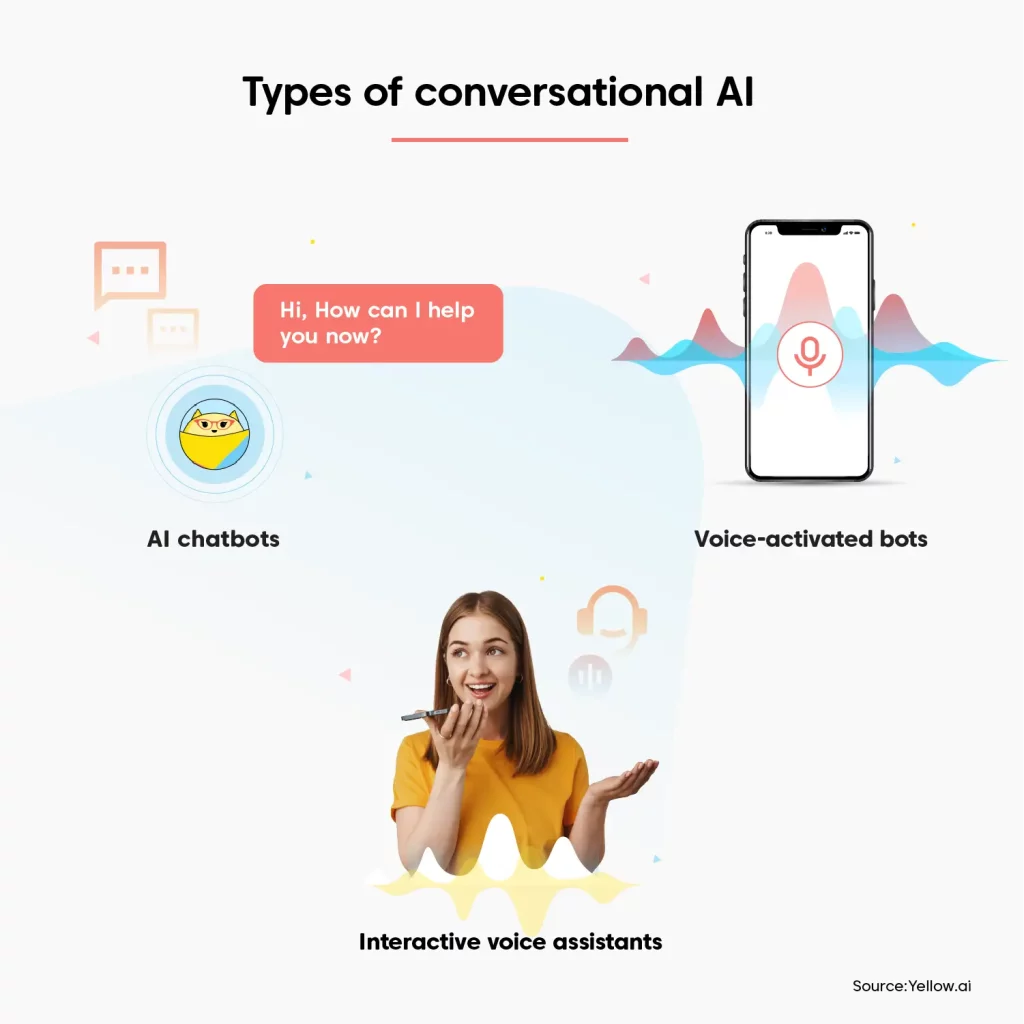
1. AI chatbots
AI-powered chatbots are software programs that simulate human-like messaging interactions with customers. They can be integrated into social media, messaging services, websites, branded mobile apps, and more. AI chatbots are frequently used for straightforward tasks like delivering information or helping users take various administrative actions without navigating to another channel. They have proven excellent solutions for brands looking to enhance customer support, engagement, and retention.
2. Voice-activated bots
Voice bots are AI-powered software that allows a caller to use their voice to explore an interactive voice response (IVR) system. They can be used for customer care and assistance and to automate appointment scheduling and payment processing operations.
3. Interactive voice assistants
Interactive voice assistants (IVAs) are conversational AI systems that can interpret spoken instructions and questions using voice recognition and natural language processing. IVAs enable hands-free operation and provide a more natural and intuitive method to obtain information and complete activities.
Conversational AI vs. chatbots: What’s the difference?
Traditional chatbots can only perform restricted activities, such as understanding and responding to user input, using pre-written replies and rules-based algorithms. Chatbots are widely utilized for customer service and assistance, answering basic questions, and completing transactions. Websites, social media, mobile apps, and messaging services can all incorporate chatbots.
AI-powered chatbots and more sophisticated systems are included in the larger category of conversational AI. Chatbots need conversational AI to enhance their capacity to comprehend human language, provide more individualized two-way user interactions, and fulfill the expectations of modern clients.
Main challenges with traditional chatbots
Although conversational AI has the potential to transform the way we interact with technology, there are still several limitations that must be addressed. Let’s look at some of the main challenges in conversational AI.
Natural language understanding and processing
Understanding and handling natural language is one of conversational AI’s significant issues. Because natural language is intricate and subtle, it can be challenging for systems to comprehend and interpret it correctly. The meaning behind the words and the conversational context must be understood by NLP algorithms, which can be challenging.
Contextualizing and keeping a coherent conversation with AI
Maintaining coherence in a discussion and comprehending the context of the dialogue are challenges for conversational AI. The context and the user’s purpose can vary over conversations, making it challenging for the system to comprehend what the user requests. It’s also difficult for the algorithm to recognize when a chat has ended and when it hasn’t.
Recognizing and managing multiple intents
During real-world conversations with AI chatbots, users can communicate several intentions or aims in a single statement. A user can, for instance, request both information on a product and a discount. As conversational AI systems must be able to comprehend and address all user requests, identifying and managing diverse intents can be difficult.
Managing ambiguity and determining the user’s genuine intent
Natural language is frequently ambiguous. It can be construed in various ways, making it difficult for conversational AI to manage ambiguity and ascertain the user’s true purpose.
Conversational AI systems frequently employ methods like natural language understanding, context modeling, and machine learning to overcome these difficulties by attempting to deduce the user’s true purpose from their words and actions.
Generating replies that are genuine and interesting
Creating authentic and engaging replies is one of the fundamental difficulties with conversational AI. The system must be able to produce replies that are accurate and helpful while still sounding natural and human-like. This is a challenge since the system must comprehend the conversation’s context and the user’s intent to reply appropriately.
Why is conversational AI important and its benefits
In today’s digital first world, conversational AI is becoming increasingly important in everyday lives. Some key reasons why conversational AI is crucial for success for customer-focused businesses – improved and around-the-clock customer service, increased efficiency, enhanced accessibility, hyper-personalization, and cost-effectiveness. In short, conversational AI is revolutionizing the way humans interact with technology, making it more intuitive, accessible, and efficient.
Some advantages of conversational AI include lower operational costs, minimal handling times, higher output, and dramatically better customer service. Let’s examine some of conversational AI’s most significant benefits and how this technology can help companies remain competitive.
Automate customer service and support tasks
One of the key advantages of employing conversational AI is the capability to automate customer care and support. Chatbots and virtual assistants can answer frequently asked questions, including giving details about goods and services, tracking orders, and resolving technical problems. This enables companies to offer customer service around the clock and enhance the customer experience.
Amplify user engagement and personalize conversations at scale
The capacity to increase user engagement and deliver individualized experiences is another advantage of conversational AI. Conversational AI systems can comprehend the user’s intent and context by utilizing natural language processing and machine learning, and they can also deliver tailored responses. As a result, the customer experience is more personalized and satisfying.
Gather insightful information about user behavior
Another advantage of conversational AI is the capacity to obtain necessary information and insights into user behavior. Companies can learn important information about their customers’ requirements, preferences, and pain points by monitoring user interactions and the conversational AI system. This data can be used to enhance goods and services and target marketing campaigns more effectively.
Lower customer service expenses and response times
Both costs and efficiency can be improved by using conversational AI. Companies can reduce labor costs and increase efficiency by automating customer service and support tasks. Furthermore, conversational AI systems can manage many customer interactions simultaneously, enabling companies to scale their operations and cater to more customers.
Increase opportunities for growth and innovation
Using conversational AI can also open up new doors for growth and innovation. Conversational AI will open up previously unserviceable use cases and applications as technology develops further. New company models, revenue sources, and growth prospects can result from this.
How a conversational AI platform can help
With the use of a conversational AI platform, the challenges described above can be reduced dramatically. Conversational AI platforms speed up AI model testing and training.
Conversational AI platforms reduce the high volume of incoming calls by automating repetitive calls so your customer support agents can focus on complex queries leading to cost savings and productivity. A sophisticated conversational AI platform will offer no-code solutions with ready-made drag-and-drop components that can assist you in quickly creating a chatbot.
Additionally, conversational AI platforms offer user analytics and monitoring to understand how users engage with the AI model, pinpoint areas for improvement and continuously enhance user experience.
Examples of conversational AI
Some examples of conversational AI are chatbots and virtual assistants like Alexa, Siri, Google Assistant, Cortana, etc. These assistants understand natural language and user intent to offer personalized responses.
Unlike traditional chatbots capable of delivering pre-built answers to questions asked in specific ways, conversational assistants understand natural language and user intent to offer personalized responses to resolve complex customer queries. Some examples of conversational AI are:
- AI chatbots example: Many companies employ AI chatbots like Yellow.ai to provide customer care and assistance. Chatbots are commonly encountered on various channels, including eCommerce sites, social media, and even through popular messaging applications like Facebook Messenger and WhatsApp.
- Voice-activated bots example: Voice-activated bots are connected to IVR systems that are triggered by user input for use cases ranging from requesting invoices and purchase history to delivering information through FAQs. A common example you may have encountered is the IVR systems that banks employ to let consumers manage their accounts, including checking account balances, paying bills, and reporting lost or stolen credit cards.
- Interactive voice assistants example: Amazon Alexa, Google Assistant, Apple Siri, and Microsoft Cortana are notable examples of interactive voice assistants which are capable of human-like conversation flows over voice channels.
Conversational AI use cases
There are several possible business applications for conversational AI. Let’s look at some of the most exciting uses of conversational AI companies use to engage customers and enhance workflows using human conversations with AI.
- Customer support automation: Conversational AI can automate customer care jobs like responding to frequently asked questions, resolving technical problems, and providing details about goods and services. This can assist companies in giving customers service around the clock and enhance the general customer experience.
- Customer engagement automation: Conversational AI can increase customer engagement by offering tailored experiences and interacting with customers whenever, wherever, across many channels, and in multiple languages.
- Conversational commerce automation: Automating tasks associated with conversational commerce, such as making product suggestions, responding to consumer inquiries, and completing transactions, is possible with conversational AI. Companies may benefit from this to enhance the customer shopping experience and boost sales.
- HR automation: Using conversational AI, HR tasks like interview scheduling, responding to employee inquiries, and providing details on perks and policies can all be automated. HR departments can do this to save time and improve the employee experience.
- ITSM automation: Additionally, conversational AI may be employed to automate IT service management duties, including resolving technical problems, giving details about IT services, and monitoring the progress of IT service requests.
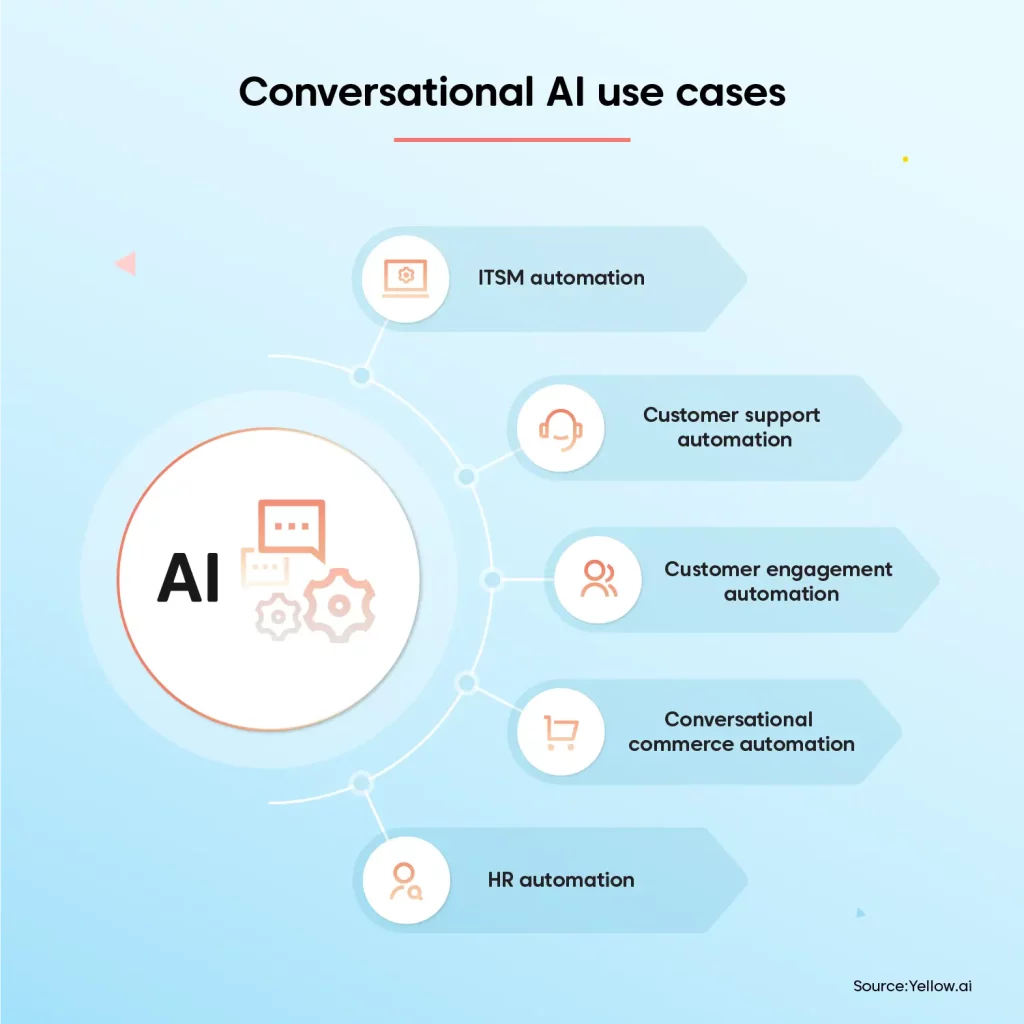
How to get started with conversational AI
Now that you have a thorough grasp of conversational AI, its benefits, and its drawbacks, let’s explore the steps you can take to introduce conversational AI into your organization immediately.
Consider your company’s long-term objectives
The first step in implementing conversational AI is considering your company’s long-term goals. What do you want to achieve using conversational AI? How do you envision it helping your company?
Defining your long-term goals guarantees that your conversational AI initiatives align with your business strategy. Make sure you ask the right questions and ascertain your strategic objectives before starting.
Identify your target audience
Once you’ve determined your long-term goals, the next step is to pinpoint your target audience. Who will communicate with your conversational AI system? What are their preferences and needs?
Employees, customers, and partners are just a handful of the individuals served by your company. Understanding your target audience can assist you in designing a conversational AI system that fits their demands while providing a great user experience.
Begin with the end in mind
Get your conversational AI system off to a strong start with a clear objective in mind. What do you want your conversational AI system to achieve? What types of interactions would you like to enable? What kind of information are you hoping to offer? By providing answers to these questions, you can create a clear vision for your conversational AI system and ensure that it fulfills your long-term objectives.
Choose the right platform
Once you have a clear vision for your conversational AI system, the next step is to select the right platform. There are several platforms for conversational AI, each with advantages and disadvantages. Select a platform that supports the interactions you wish to facilitate and caters to the demands of your target audience.
You can also partner with a next-gen total experience automation platform such as Yellow.ai to create a no-code, AI and DynamicNLP™ powered, multilingual chatbot in just a few clicks.
Start building your first bot
Once you have decided on the right platform, it’s time to build your first bot. Start with a rudimentary bot that can manage a limited number of interactions and progressively add additional capability. Test your bot with a small sample of users to collect feedback and make any adjustments.
Continuously evaluate and enhance your bot over time to guarantee that it achieves your long-term goals and offers a great user experience.
Improve your customer experience with the right conversational AI tools
Conversational AI can improve the customer experience by offering a natural and intuitive approach for customers to connect with a company. Customers can obtain support, accomplish activities, and get the information they want in a conversational way, increasing user satisfaction and engagement. Conversational AI is becoming increasingly potent and valuable due to machine learning, context modeling, and natural language comprehension developments.
If you want to improve your customer experience, look no further than Yellow.ai’s conversational AI platform. Yellow.ai provides a comprehensive set of tools and capabilities to assist you in developing productive and compelling conversational AI experiences. Request a demo today to see how conversational AI can help your company and customers.