Conversational AI is bridging the gap between users and businesses by providing a seamless customer and employee experience. What started out as simple FAQ-based chatbots are today helping enterprises with:
- HR and ITSM automation for employees
- 24/7 customer support automation with intelligence
- Sales-driven conversational commerce
- Customer marketing for increasing engagement and awareness
Despite the many benefits, implementing and getting started with the conversational AI platform comes with its own set of challenges. Conversational AI platforms need considerable training to effectively provide human-like conversations. According to a survey conducted by Deloitte, setup challenges, including training data and maintenance, were among the top reasons for not implementing chatbots in enterprises.
NLP model training is indeed a time-consuming, tedious and complex process. We are truly excited to announce Yellow.ai’s DynamicNLP™, a first of its kind innovative technology solution in the enterprise conversational AI market that completely eliminates the need for training the NLP model. Yellow.ai DynamicNLP™ is developed based on the cutting-edge Zero-shot learning that ensures enterprises no longer need to go through the lengthy process of manually labeling data to train the bot.
Let’s take a deeper look into this.
What is Yellow.ai’s DynamicNLP™?
Yellow.ai DynamicNLP™ is at the cutting edge of technology: Zero-shot learning. The platform comes with a pre-trained model with unsupervised learning that operates right out of the box. This means there is no need to spend time training your own model manually, and you can go live much faster within minutes with higher intent accuracy.
“Yellow.ai is making Conversational AI simple and accessible for everyone,” said Pranshu Rastogi, Head – Customer Experience & AVP – Marketing, Domino’s. “With the new DynamicNLP™, Yellow.ai is making it possible for businesses to easily add sophisticated NLP capabilities to their applications without any specialized expertise or lengthy setup times, because there’s no more NLP model training required. This is indeed a breakthrough innovation, many congratulations to Yellow.ai for bringing research to the hands of the businesses.”
- Zero-shot learning
Yellow.ai DynamicNLP™ is based on cutting-edge technology Zero-shot learning, allowing businesses to bypass the tedious, complex and error-prone process of model training. - Pre-trained model with unsupervised learning
Our NLP engine uses an unsupervised learning approach, which completely eliminates the need for training NLP with data points and helps to up the conversational AI platform within minutes. - State-of-the-art NLP engine built after ingesting billions of conversational data
Our NLP has “seen” all different syntactic variations of sentences from billions of conversational data. This allows you to deliver a seamless customer experience with a better understanding of the context and intention of their queries. - Accurate understanding of intent from day one
Continuous upgrade to the NLP engine helps the Dynamic AI Agents improve the intent performance, which ensures that your customers get accurate responses from day one.
Traditional vs. Yellow.ai DynamicNLP™
The main idea behind Yellow.ai DynamicNLP™ is that you can create “intents” without NLP training and take those intents live. To showcase how DynamicNLP™ works, let’s take the example of an airline named SkyFly.
SkyFly wants to provide automated customer support with the conversational AI platform. One use case that they are looking to automate is “Get flight status.” So whenever a customer asks the chatbot about the flight status, the chatbot will provide the live update based on the flight number.
Traditional workflow without Yellow.ai DynamicNLP™
Without Yellow.ai DynamicNLP™, designing the conversational flow for this use case would require the following steps:
Step 1: Create intent named “Get flight status”
Step 2: SkyFly then would have to list all the utterances related to this intent.
For example: It would create training data such as “Flight status,” “What is the arrival time for my flight,” “Is my flight on time,”, “Is there a delay in 6W -1234,” and so on for this single use case.
The biggest challenge in this process is to think ahead of time: How are my customers going to ask about their flight status?
It may be easy to identify a few utterances like “flight status,” “what is my flight status,” etc., but these cover only a fraction of all the possible queries the customers might ask with respect to fetching their flight status.
Step 3: Someone will have to manually feed all the training data/utterances in the platform.
Step 4: Train and test the model.
Step 5: Keep repeating the entire process to add each unidentified utterance by the customers while the bot is live.
Also note that we have considered the single intent “Get flight status.” There will be hundreds of intents against which model will have to be trained.
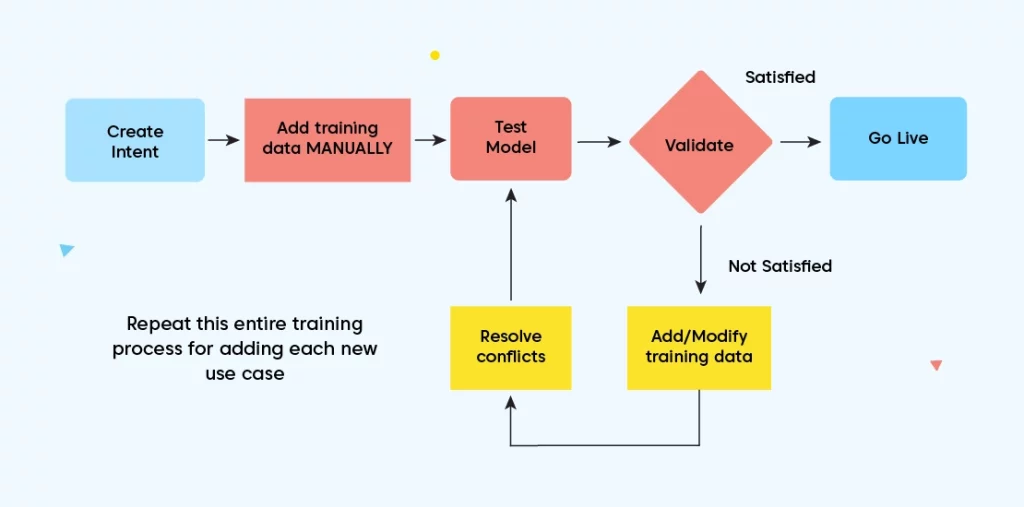
Drawbacks of this supervised approach
- Repetitive process to improve accuracy: With the supervised approach, the right way to add utterances would be to constantly monitor how your users are typing utterances and then incrementally and iteratively label the ones that did not get identified. Once labeled, add these missed utterances back to training. This means that there will be customers whose queries would have gone unidentified in this process. They would have interacted with the bot and did not get the help. Is this the kind of frictionless experience we would want to give to our customers? Definitely NOT!
- Long tail queries: Another big challenge with this workflow is the coverage and the extent to which utterances can be added. Even if all the different ways in which users can ask for flight status are taken into consideration, how many of those will the chatbot be able to pick up?
For instance, if a customer asks: “I have booked a flight to Amsterdam for the date Aug 20. I want to check the list of other flights that I can update my booking with for the date Aug 21.” This kind of long tail query will generally fail because the model does not have enough coverage of the “long tail”. The only way to improve a chatbot is by routinely monitoring conversations and adding the utterances that fall back to training. - Time-consuming process: For the conversational AI platform to function at its best, the platform would need to be continuously trained and maintained. Unless someone keeps adding and updating utterances, the platform will fail to understand users’ intent and fail to have human-like conversations. And this needs to be maintained for tons of use cases, meaning manually training NLP with hundreds to thousands of data points.
- Expensive: NLP training is a costly process. Human resources need to be employed to train the model and it takes time to manually feed the training data. Companies are missing the great opportunity of customer connect during the training time when the conversational AI platform is not live or is failing and they are losing out on the business.
Yellow.ai DynamicNLP™ workflow
With Yellow.ai DynamicNLP™, we have leveraged unsupervised machine learning methods to train the NLP model, without the need for manual labeling.
Now to automate the “Get flight status” use case, all SkyFly needs to do is create an intent named “Get flight status.” The Yellow.ai DynamicNLP™ engine will automatically learn from this intent name and work for user messages related to this intent. So there is no need to think ahead of time to manually add all the utterances. Moreover, it continues to learn in real-time and evolve dynamically.
What’s the logic behind this magic?
By now, you have probably figured out that for highly accurate intent understanding you would need large quantities of high-quality labeled data. But getting high-quality data and manually feeding it in NLP is hard, very hard. Yellow.ai DynamicNLP™ works on the cutting-edge technology Zero-shot learning. With Zero-shot learning, our model learns how to classify classes/intents that it has not seen before, without the need for NLP training.
Let’s simplify this: The model comes with a pre-trained NLP from billions of conversations across industries. When SkyFly creates an intent named “Get flight status,” there is no need to explicitly train the bot, because the model already has a good understanding of all the utterances related to “Get flight status.”
Technically behind the scenes, when a customer asks “What’s the arrival time of the flight from San Francisco to Chicago?”, Yellow.ai DynamicNLP™ generates the sentence embedding for the intent: What’s the arrival time of the flight from San Francisco to Chicago? It then uses all the learning across the billions of conversation data and picks the intent that has the highest match, in this case intent named “Get flight status.” This way there is no dependency on training data. No one needs to manually feed the training data/utterances for effective natural conversations. And from day one itself, the model has stronger NLP and better intent accuracy.
Benefits of Yellow.ai DynamicNLP™
- Better coverage: Most off-the-shelf sentence embedding models are trained on generic language data like news articles, Wikipedia articles, etc. The way people type into chatbots is very different from how language is structured in these publicly available articles. In other words, conversational language is not the same as that in articles. The Yellow.ai DynamicNLP™ model has “seen” all different syntactic variations of sentences from billions of conversational data and hence would generate stronger embeddings for chatbot settings.
- Improved intent accuracy across industries: Yellow.ai DynamicNLP™ is exposed to a lot of domain-related utterances. This means that the subsequent sentence embeddings that are generated are much stronger with 97%+ intent accuracy. With Yellow.ai DynamicNLP™, a 20–60% reduction in unidentified utterances is possible depending on the industry.
- Go live in minutes rather than months: Traditionally with the supervised approach, just to train the model for 10 use cases would require a minimum of 100–200 utterances that would have to be manually added. Yellow.ai DynamicNLP™ is based on an unsupervised Zero-shot learning algorithm, meaning no need to manually add the training data. Enterprises can now go live faster with the conversational AI platform as the time to manually add all those utterances is no longer needed.
- Cost-effective and increased deflection to improve the ROI of conversational AI platform: With Yellow.ai DynamicNLP™, there is no need for training the NLP model. ML scientists wouldn’t need to work on mundane data clean and data prep and help them focus on deep learning workflows. In addition, with Yellow.ai DynamicNLP™, enterprises can go live within minutes rather than months as the initial training time is not wasted. And finally, Yellow.ai DynamicNLP™ provides better intent coverage across industries. So there are more customers whose queries get identified resulting in higher automation. This leads to better customer experience as there is quick and accurate resolution of their queries with increased deflection rate.
Empowering enterprises with Yellow.ai DynamicNLP™
Yellow.ai DynamicNLP™ based on Zero-shot learning allows the model to learn from the intent name itself. This means that the model can learn on the fly, without needing to be trained on each new domain.
Commenting on the launch, Puneet Kaur Kohli, CTO, Universal Sompo General Insurance Co. Ltd., said “Yellow.ai’s DynamicNLP™ is another step towards helping businesses get started with their chatbot deployments quickly and without any hassle. With this innovation, businesses can now easily create and deploy intelligent chatbots across channels such as Facebook Messenger, WhatsApp, WeChat, Twitter and many others. DynamicNLP™ is a natural language processing (NLP) engine that understands user intent by constantly learning from billions of real-world conversational data points. It enables businesses to not only quickly build chatbots but also accurately interpret what users are asking for – making customer interactions more efficient and delightful.”

Faster and easier deployment of conversational AI platform
- Go live faster by reducing months of development efforts and model training
- Set up conversational AI flows within minutes and not weeks!
Cost reduction in the implementation of the platform
- Lower operational cost by eliminating the need for manual labeling of text and training the bot
- Save time and effort with zero NLP training
Enterprise-ready to power up your automation from day one
- First in enterprise conversational AI space
- Increase in intent understanding with over 97% accuracy
Save your spot
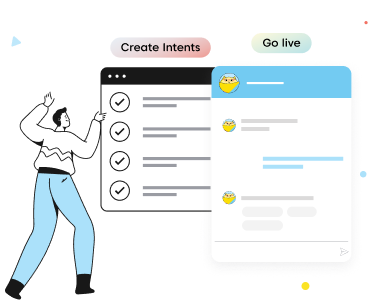